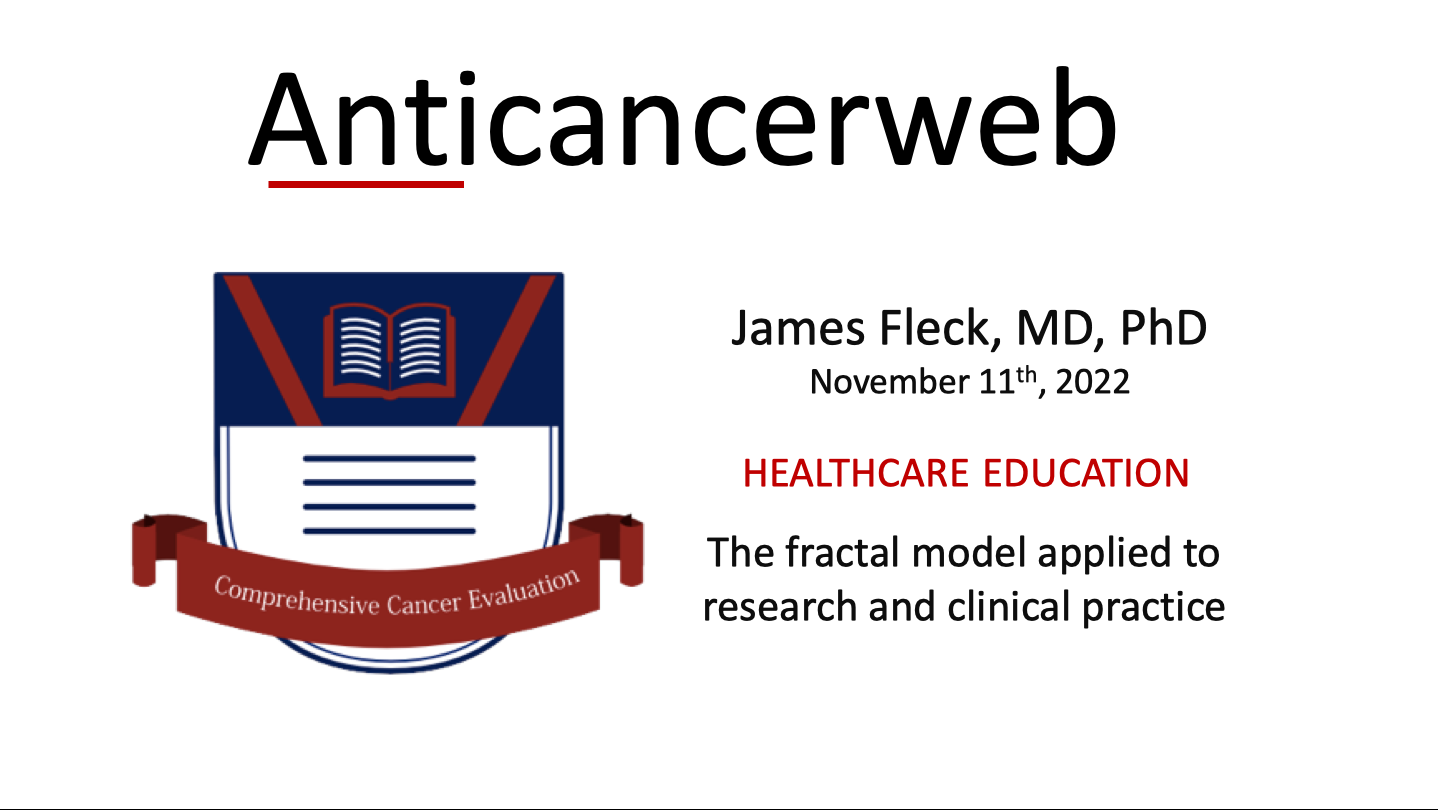
Medical science will be predictable in the near future
James Fleck, MD, PhD
Nowadays, randomized controlled trials (RCT) are the cornerstone of medical science. They represent the highest level of evidence to support diagnostic and therapeutic advances. In the history of clinical research, the first RCT was designed in 1946 and chaired by Sir Geoffrey Marshall assisted by two statisticians named Sir Austin Bradford Hill and Philip Hart. The trial was initiated in 1947, evaluating the use of streptomycin in the treatment of pulmonary tuberculosis. Here, Dr Hill instituted randomization, a new statistical process which has been further described in a landmark article published in the British Medical Journal. The central issue, in the randomization program proposed by Dr. Hill, was allocation concealment, replacing the alternating distribution of patients by a new masked strategy, using a set of sealed envelopes, each containing only the hospital name and a number. This technique avoiding selection bias, gradually increased in sophistication through the use of new randomization criteria, including block, stratified or covariate adaptive methodology. For more than 75 years, advances in medical science have been based on random selection, responsible for the unpredictability of outcomes. Medicine became a science supported by statistics, and physicians used to explain their recommendation on the basis of probabilities. RCT and meta-analysis are now used as the best evidence to propose a new standard in disease’s diagnosis and treatment. However, despite the rigorous methodology, standardization does not perfectly match the random process, as it will never include patients with exactly the same risk factors and biological disease clinical and microenvironment behavior. Currently, clinical guidelines are based on the level of evidence and recommendations supported by peer review of the literature, contextualization and consensus. It is a kind of best-fit model, using a heuristic problem-solving methodology.
Cancer is the second leading cause of death in the world. Let's use cancer as a model of reasoning. In the last fifty years, RCT and meta-analysis have created almost the entire evidence-base to support advances in cancer prevention, diagnosis and treatment. Currently, both oncology research and practice have progressively been sustained in precision medicine. A huge amount of data has been obtained from several molecular signaling pathways. All of them are represented by descriptive metaphoric illustrations. Figure 1 shows tyrosine-kinase downstream signaling pathway, one of the various mechanisms associated to carcinogenesis. Here, a communication network is represented at the molecular level and is used to test new targeted therapies, often using RCT and meta-analysis. A similar approach is used in other signaling pathways and in current cancer immunotherapy (blockade of immune checkpoints). However, even in these sophisticate scenarios there are limitations for RCT. The main one is the need to analyze homogeneous populations, assuming that damage is occurring in only one signaling pathway at a time. However, this is a simplistic and artificial view, as is its metaphorical description. Living organisms are dynamic and microenvironmental behavior is dependent on a connected signaling network. In the microenvironment of tumor cells there is phenotypic plasticity, which extends to molecular, genomic, morphological and functional levels. This explains the early recurrence and high level of drug resistance in metastatic disease.
Figure 1: Activation of HER receptor and dimerization: (A) Structure of HER receptor: extracellular realm containing four domains (I,II,III and IV) + helical transmembrane segment + intracellular protein tyrosine kinase domain, (B) Spatial conformation of HER receptor, exposing the ligand binding domain I and III, (C) Ligand binding, activating HER receptor, (D) Dimerization leading to the proximity of the c-terminal of tyrosine kinase domains after homodimerization or heterodimerization and phosphorylation.
Recently, The Cancer Genome Atlas (TCGA) represented a more integrative methodology. TCGA molecularly characterized more than 20,000 primary tumors, matching normal samples in 33 common cancer types. TCGA has six different comprehensive platforms, compiling clinical, genomic, epigenomic and proteomic data. All platforms are supported by increasing database and can be visually represented. Putting in perspective, this is the beginning of a new era, hopefully sustaining a more accurate methodology to support clinical decision-making. Integrating effort of well-designed knowledge graph and artificial intelligence resources can hopefully identify patterns, both for normal and progressive disease stages. What happens in cancer reasoning model can be extrapolated to other scenarios. These advances would make medical science more predictable in a near future.
Let's momentarily combine science and philosophical reasoning, assuming dialectical interaction as a constructive learning process. Let's start from the premise that medical science can be completely accurate and predictable. What model could best describe biological systems, both in health and disease? Well, almost everything in nature is based on fractal geometry. The human body is entirely composed of fractal geometry. These is observed both in body morphology and physiology. The bronchial tree is composed of the same fractal dimension until the seventh-generation branch and then goes to a higher dimension. Liver, kidneys, brain and pancreas are also organs that can morphologically be described along the same self-similarity fractal rule. Heartbeats follow fractal pattern. Blood flow is fractal. What is very interesting is that cerebral blood flow increases in areas where there is greater neuronal activity. The increasing use of functional magnetic resonance imaging of the brain may evoke fractal patterns in cognitive, emotional and functional activities in both health and disease. Figure 2 shows a Golgi stained Pyramidal neurons from the human visual cortex, using magnification 100X. Making this vision more thought-provoking, the image resembles Jackson Pollock's Summertime: Number 9A, painted in 1948. The artist never studied biology, but he firmly believed that the painting represented the energy and movement of inner forces. Like the neurons in the human visual cortex, Jackson Pollock's work has patterns that resemble fractal geometry, which may indicate an expression of human art oriented both emotionally and physiologically. In nature, behavior can also be supported by fractal patterns. Maybe the best example is the mathematical model observed in the flight of the starlings, a phenomenon called murmuration, due to the elicited sound. It’s a collective expression of movement and noise, sustained by individual signaling code, orienting the entire group. This phenomenon, might resemble the molecular signaling models expressed in the human body microenvironment. Next generation sequencing advances in technology will allow identification of most of DNA variants, including insertions, deletions, duplications, inversions, repeat expansions, and others, which will reflect in the clinical expression of the diseases. Interestingly, a cluster computer nucleotide sequence by fractal analysis has been recently described, expressing a high predictive value.
Figure 2: Fractal dimension in Golgi stained Pyramidal neurons from the human visual cortex (A), Jackson Pollock’s Summertime: Number 9A (B), flight of the starlings murmuration (C) and DNA Next-Generation Sequencing (D)
Cancer microscopic cell arrangements, immunohistochemistry and molecular phenotype can be described on fractal patterns. Fractals are defined by various levels of organization, irregular shapes, and self-similarity. Fractal geometry deals with non-integer dimensions, where each part of the image is similar to the entire image. In general, when phenotypic expression changes from a normal to a diseased state, a sudden jump to a higher fractal dimension occurs. But still, a pattern can be identified and eventually predicted. But, this advance in knowledge is entirely dependent on advances in artificial intelligence. Fractal geometry concept was first described by Benoît Mandelbrot. In 1980, the mathematician moved to IBM, where he used complex numbers and quadratic polynomials to build an infinite iteration image. This image, known as Mandelbrot set, was the first one to reproduce, in an electronic system, the fractal geometry found in nature. In fractals, despite the irregularity, complexity and non-linearity, there is a pattern that is perceived and recognized and that can be predicted. Little in fractal computer modeling has been done after the Mandelbrot set. Some attempts began to emerge in clinical research with small and very specific use in patient care. The main reason is the difficulty of identifying irregular and dynamic patterns both in health and disease. But, they are there, waiting to be found by AI. This might sound like flying over fiction! Actually, AI will never surpass human brain creativity, but it certainly is a very useful tool for working with big data. Physicians and computer science engineers should cooperate in developing a new ontology to communicate medical data globally. The generated big data would be progressively used to produce knowledge graphs, better feeding machine-learning algorithms. The result could be the increasing identification of specific fractal patterns in human body. As the fractal dimension is predictable, patterns would lead medical science to more precise and effective interventions.
References:
1. MRC Streptomycin in Tuberculosis Trials Committee. Streptomycin treatment of pulmonary tuberculosis. BMJ 2:769-83, 1948
2. Ann Bowling: researches Methods in Health: investigating Health and Health Sciences, Open University Press, McGraw Hill Education, Berkshire, England and Two Penn Plaza, New York, USA, 4th edition, 2014
3. B Mandelbrot: How long is the coastline of Britain? Statistical self-similarity and fractal dimension, Science 156: 636-638, 1967
4. G Landini and JW Rippin: Fractal fragmentation in replicative systems, Fractals 1: 239-246, 1993
5. L Goldberger: Non-linear dynamics for clinicians: Chaos theory, fractals and complexity at the bed side, Lancet 346: 1312-1314, 1996
6. JW Baish and RK Jain: Fractals and Cancer, Cancer Research 60, 3683-3688, 2000
7. E Sabo, A Boltenko , Y Sova, A Stein, S Kleinhaus, MB Resnick: Microscopic analysis and significance of vascular architectural complexity in renal cell carcinoma, Clin Cancer Res 7(3):533-7, 2001
8. N Lesmoir-Gordon, W Rood and R Edney: Introducing fractal geometry, Icon Book, UK, 2003.
9. P Dey: Basic principles and applications of fractal geometry in pathology: a review, Anal Quant Cytol Histol 27(5):284-90, 2005
10. K Metze: Fractal dimension of chromatin: potential molecular diagnostic applications for cancer prognosis, Expert Rev Mol Diagn, 13(7): 719 – 735, 2013
11. J N Weinstein, E A Collisson, G B Mills, et all: The Cancer Genome Atlas Pan-Cancer analysis project, Nature Genetics 45(10): 1113-1118, 2013
12. DM Mathews and KSM Panicker: Cluster Computing of Nucleotide Sequence by Fractal Analysis, International Journal of Scientific & Engineering Research, Volume 5, Issue 2, 2014
13. J-S Lee: Exploring cancer genomic data from the cancer genome atlas project, BMB Rep 49(11):607-611, 2016
14. S Kisan, S Mishra, S B Rout: Fractal Dimension in Medical Imaging: A Review, International Research Journal of Engineering and Technology 4(5): 1103-o6, 2017
15. I A Mavroudis, F Petrides, D Kazis, V Costa and S Baloyannis: Golgi Method: A 140 years old yet unique and powerful method for the study of central nervous system, Academy of Romanian Scientists Annals - Series on Biological Sciences, Vol. 6, No.1, 2017
16. F Sanchez-Vega, M Mina, J Armenia, et al: Oncogenic Signaling Pathways in The Cancer Genome Atlas, Cell 173(2): 321-337, 2018
17. Jackson Pollock: Summertime, Number 9A, Tate Gallery, 1948
18. A G Lorente: Mathematical Modeling of the Flight of the Starlings. A Particular Case of an Attractor Repeller Pairs, Universal Journal of Computational Mathematics 7(1): 14-20, 2019
19. Next-Generation Sequencing Challenges: NGS growing by leaps and bounds, problems arise, Genetic Engineering Biotechnology News, 2017
20. J Fleck: Her-2 dual blockade strategy in the curative treatment of early breast cancer, Anticancerweb 17 (03), 2021
21. L G da Silva, M da Silva, T M Aguiar Moreira, et al: Fractal dimension analysis as an easy computational approach to improve breast cancer histopathological diagnosis, Applied Microscopy 51: 6, 2021
22. O Campbell, T Vanderwal and A M Weber: Fractal-Based Analysis of fMRI BOLD Signal During Naturalistic Viewing Conditions, Frontiers in Physiology: Volume 12, Article 809943, 2022
23. L Elkington, P Adhikari, P Pradhan: Fractal Dimension Analysis to Detect the Progress of Cancer Using Transmission Optical Microscopy, Biophysics 2 (1): 59 – 69, 2022
24. SB SinoBiological: (www.sinobiological.com)
25. J Fleck: Global e-PHR (www.ephr.org)
The way of onocologyst work is changing. We are coming to era when we gonna say to patient '' we gonna cure your cancer, you have to take this pill for X mouths, don't need to chemotherapy'' I belive this.
The rationalization of medicine is an extensive process, for hundreds of years seeks patterns that justify the process of the disease and also its treatment. The fractal view of the area exposed in the article is the maximum expression of this long walk, which seeks to lay on firm, precise and scientific bases the secular medical knowledge in the search for maximum efficiency and in the concomitant escape of biases.
It is interesting to think that behaviors are based on probabilities, and the rct are essential today for rational and scientific thinking. The article describes the evolution of this scientific thinking. The RCT revolutionized the scientific method in medicine, but we know that it has its flaws and we always have to seek innovation and improvement of our methods, seeking new patterns, such as the fractal pattern described in the article.
It’s important for the advance of medical science that we use more sophisticated methodologies in our studies, in order to account for the countless interactions involved in malignancies. Investigating their fractal geometry through modern data science techniques (AI included) is likely to produce much better models for predicting cancer development and morphology, resulting in advances to precision diagnosis and treatment. It may also give us new insights on the mathematical patterns present normally in humans. However, it may be impossible to produce a perfect deterministic prediction of cancer, given the inherently probabilistic nature of quantum systems, including biochemical reactions.
Reading the text allows us to infer that medicine is a science in constant transformation: more than a critical observation of the medicine of the future, it is possible to remember the values inherent in medical practice. Thus, permeated by an infinity of studies and evidence that seek to justify conducts, it is up to the physician, always, the ability to offer the best possibility, respecting the principle of benefit. More than knowing the countless pathologies, the doctor must be an instrument to offer the “probabilities” and “possibilities” that involve the patient's disease, contributing to a more humane and shared practice in the oncological scenario.
The consolidation of Evidence-Based Medicine poses challenges to medical science as it requires it to become increasingly accurate and predictable. In this scenario, AI can be a major ally, helping to identify patterns that may go unnoticed to the human eye, as is the case of geometric fractals. The tendency is that the combination of human creativity with the use of technology will allow us to create diagnostic models and treatment alternatives that are increasingly individualized and precise.
The constant evolution of medicine requires doctors to offer the best treatment options while respecting the principle of benefit. Evidence-Based Medicine poses challenges to medical science, which can be addressed through the use of AI and modern data science techniques to identify patterns in malignancies, resulting in more accurate prediction models and personalized treatment. Investigating the fractal geometry of cancer may also reveal new insights on mathematical patterns in humans. However, due to the probabilistic nature of quantum systems, including biochemical reactions, it may be impossible to produce a perfect deterministic prediction of cancer.
The article highlights the importance of acknowledging the limitations of the current medical approach to cancer treatment and embracing a patient-centered, holistic approach that takes into account the physical, emotional, and spiritual needs of each individual. It is crucial to move away from the idea that cancer is solely a matter of statistics and probabilities, and instead recognize the unique experiences and perspectives of each person facing the disease. This shift towards personalized care can lead to better outcomes and a higher quality of life for patients, as well as a greater sense of empowerment and agency in the face of a daunting diagnosis.
The article discusses the current methodology in medical science based on randomized controlled trials (RCT) and meta-analysis, which are used to propose new standards in the diagnosis and treatment of diseases. While these methods provide rigorous evidence-based standards, they are limited by the fact that they do not match the random process perfectly. The article uses cancer research as an example to illustrate how precision medicine and new integrative methodologies like The Cancer Genome Atlas (TCGA) are creating more accurate models to support clinical decision-making. Despite this progress, the article suggests that a completely accurate and predictable model of biological systems is not feasible, as nature is based on fractal geometry and systems are too complex to be completely understood.
The article discusses the current methodology in medical science based on randomized controlled trials (RCT) and meta-analysis, which are used to propose new standards in the diagnosis and treatment of diseases. While these methods provide rigorous evidence-based standards, they are limited by the fact that they do not match the random process perfectly. The article uses cancer research as an example to illustrate how precision medicine and new integrative methodologies like The Cancer Genome Atlas (TCGA) are creating more accurate models to support clinical decision-making. Despite this progress, the article suggests that a completely accurate and predictable model of biological systems is not feasible, as nature is based on fractal geometry and systems are too complex to be completely understood.
Although randomized clinical trials are considered the best way to establish continuous causal relationships in medical practice, it must be admitted that they have limitations, since they are unable to fully capture the real biological and environmental plurality of the randomized individuals - which explains the fact that many drugs fail even when directed to extremely specific molecular signaling pathways and supposedly implicated in the pathogenesis of the disease. Thus, recognizing the dynamics and irregularity of living organisms, for example, when trying to see reality from a fractal perspective, as well as understanding the importance of artificial intelligence in this context, is the first step in seeking greater advances in medicine.
Since randomized clinical trials have limitations and are unuble to understand some individual patient behaviors, a new form of clinical research is really needed. In this context, it's a great advence that science is evolving towards a model that can do this even more especifically and reliably, such as artificial intelligence.
One of the most interesting aspects of research in health and treatment is the racional and the empirical part envolved in new thesis around a new treatment or medicin. Both the heavy theory with physiology (racional) and the RCT (empirical) are essencial on the way research works. Within the oncology macroverse, this aspect gets stressed, along with the fast way biomolecular markers are developed.
Please login to write your comment.
If you do not have an account at Anticancerweb Portal, register now.